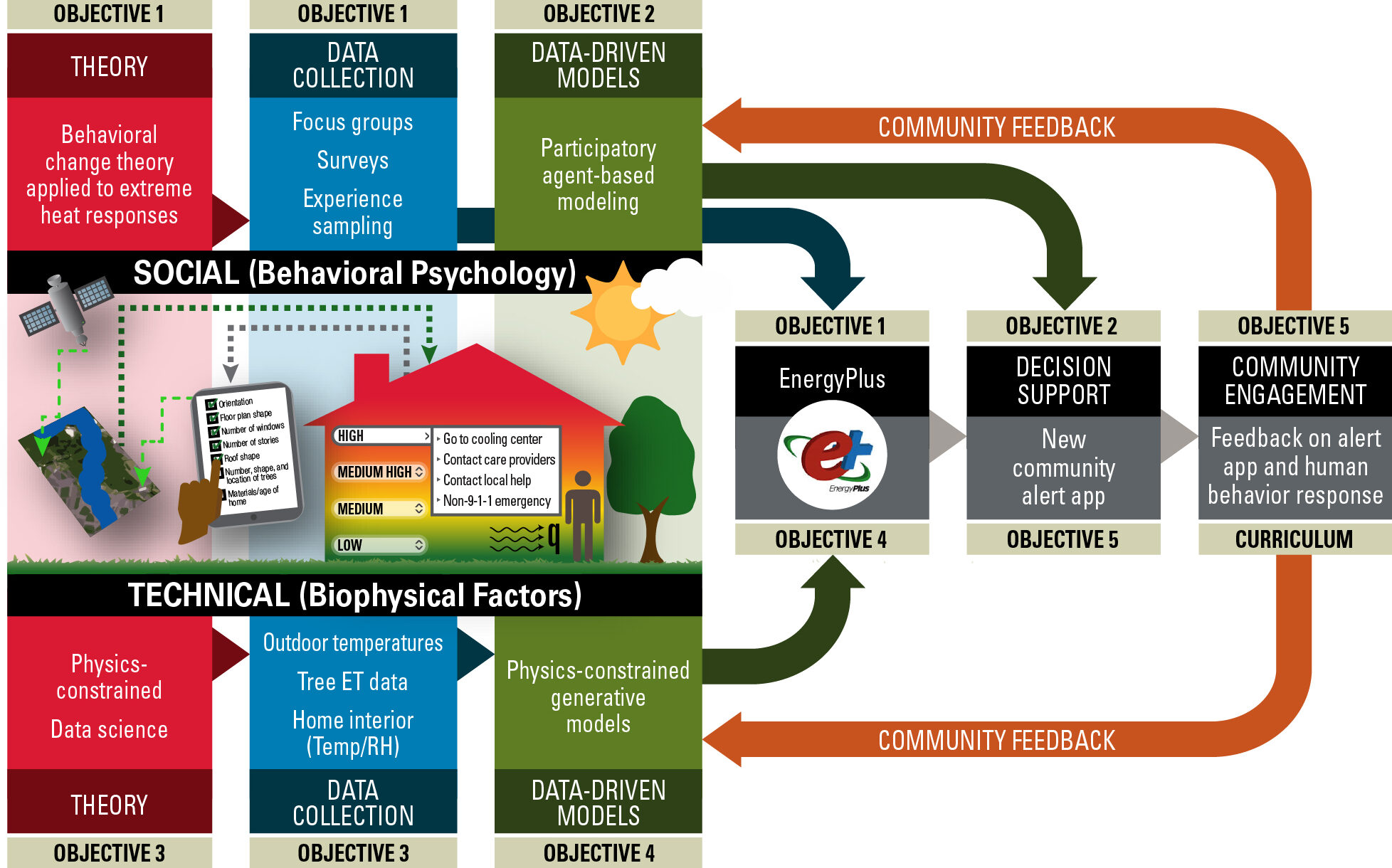
Project Challenge
Extreme heat is the deadliest type of weather in the US and disproportionately affects vulnerable populations. Called the ‘silent killer’ by health experts due to its gradual and invisible nature, extreme heat is especially dangerous for the elderly and low-income residents who are less likely to have centralized or adequate home cooling.
The 4th US National Climate Assessment predicts that extreme heat events will increase markedly with significant threat to human health. Lack of central air-conditioning and the impact of urban heat island effects caused by urban density and lack of tree canopy can create dangerous indoor living conditions, exacerbated in low-income neighborhoods.
Project Vision
The overarching goal is to develop a community-focused microclimate-informed indoor heat emergency alert (CommHEAT) system that will enhance and personalize community heat-related emergency management capacity. The framework will combine data collection from residents and community partners in the Capitol East neighborhood of Des Moines with human behavior change theory and scientific machine learning (SciML) approaches to reliably predict heat flux and human response in five integrated objectives.
Our approach is novel in its use of empirical data and participatory modeling with vulnerable community residents and stakeholders to iteratively co-design information technology for public health.
Integrative Research
CommHeat develops and evaluates a framework for engaging community members and civic officials in co-development of specific and personalized responses to mitigate effects of extreme heat, with a focus on vulnerable populations.
Using a Behavior Change Theory framework, data is collected on current adaptation strategies in extreme heat events and perceptions of HHWS from residents/community partners to define the range of adaptation and triggers. Modeled human behavior will be integrated with physics-informed SciML models. Our ability to design alerts will improve as we learn how social and microclimate conditions are mediated in urban systems.
Data-driven SciML models will increase ability to tailor alerts to specific people/situations/events, including specific locations or buildings that are at high risk given the current local environmental conditions.
Individualized, tailored alerts will result in better decision-making, provide actionable behavior distribution of temporary cooling equipment, and will lead to reduced vulnerability to thermo-physical conditions of indoor and near-building spaces. The project is well integrated with the City of Des Moines climate action and adaptation plan, ADAPT DSM.
Project Website
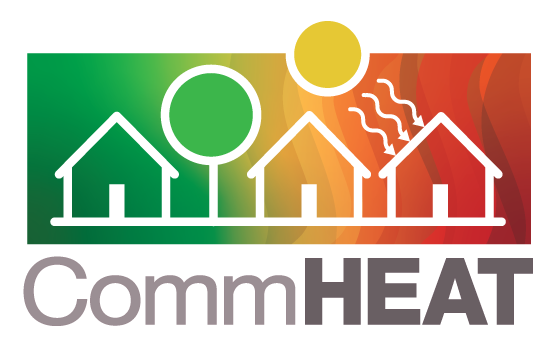
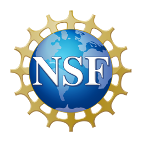
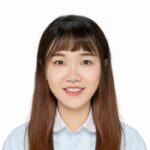
Tian Yao
PhD Student in Human Computer Interaction